What are the mathematical prerequisites for data science?
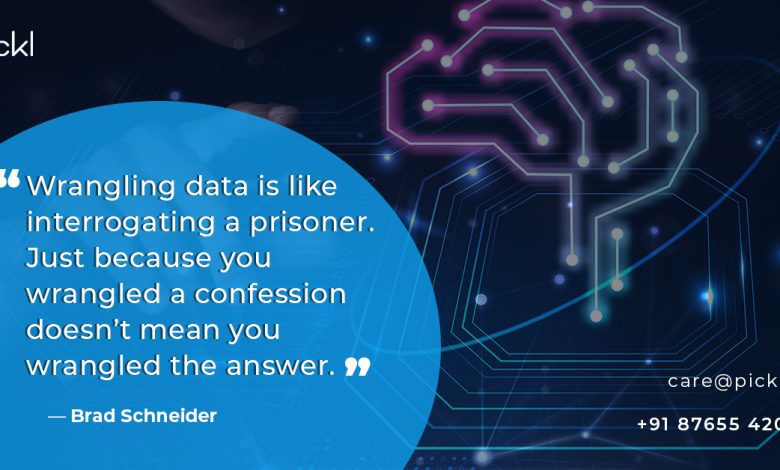
Mathematical prerequisites for data science: Math is the bedrock of any contemporary discipline of science. Practically every one of the methods of present-day information science, including AI, has profound numerical support.
It’s implied that you will require a wide range of various pearls of information about the data to work as a top data scientist. Preferably a good programming capacity, some measure of business insight, and your extraordinary logical and curious mentality.
The knowledge of fundamental math is especially significant for rookies showing up at data science from different callings. For instance in equipment designing, retail, the compound interaction industry, medication, and medical care, business executives, and so forth. Data science, by its actual nature, isn’t attached to a specific branch of knowledge. Because it may manage peculiarities as assorted as malignant growth judgments and social conduct investigation. This delivers the chance of a confounding exhibit of n-layered numerical items, factual conveyances, improvement objective capacities, and so on.
Here are my ideas for the data scientist eligibility criteria to study to be at the highest point of the game in information science.
-
Capacities, Variables, Equations, and Graphs
Where You Might Use It: To comprehend how a hunt runs quicker on 1,000,000 thing information bases after you’ve arranged it, you will run over the idea of “paired search.” To comprehend its elements, you will require knowledge of how to grasp logarithms and repeat conditions. Or on the other hand, if you need to break down a period series, you might go over ideas like “occasional capacities” and “outstanding rot.”
-
Measurements
The significance of having a strong embrace over fundamental ideas of insights and likelihood couldn’t possibly be more significant. A huge number in the field think about traditional (non-brain organization) AI to be only factual learning.
Where You Might Use It: In interviews. If you can show you’ve dominated these ideas, you will intrigue the opposite side of the table quickly. You will also involve them essentially consistently as an information researcher.
-
Direct Algebra
This is a fundamental part of science for understanding how AI calculations work on a surge of data to make use of it.
Where You Might Use It: If you have utilized the dimensional decrease strategy head part investigation, you have likely utilized the particular worth disintegration to accomplish a conservative aspect portrayal of your informational index with fewer boundaries. All brain network calculations utilize straight polynomial math procedures to address and deal with network designs and learning activities.
-
Analytics
Whether you adored or loathed it in school, analytics springs up in various spots in data science and AI. It prowls behind the straightforward-looking logical arrangement of a normal least squares issue. For example: in direct relapse or implanted in each back-engendering your brain network.
Where You Might Use It: At any point consider how precisely a relapse calculation is carried out? There is a high possibility that it utilizes a technique called “angle plummet” to find the base misfortune work. To comprehend how these function, you want to utilize ideas from math: inclination, subsidiaries, cut-off points, and chain rule.
-
Discrete Math
This region isn’t in talking as frequently in data science; however, all advance data science finishes with the assistance of computational frameworks, and discrete math is at the core of such frameworks.
Where You Might Use It: In any interpersonal organization examination, you really want to know the properties of a diagram and quick calculations to look at and navigate the organization. In any decision of calculation, you want to figure out the existence intricacy. For example: how the running reality prerequisite develops with input information size, by utilizing O(n) (Big-Oh) documentation.
-
Advancement and Operation Related Topics
These points are most applicable in specific fields like hypothetical software engineering, control hypothesis, or activity research. However, a fundamental comprehension of these strong methods can likewise be productive in the act of AI. Essentially every AI calculation intends to limit some sort of assessment blunder subject to different requirements — which is an improvement issue.
Where You Might Use It: Straightforward direct relapse issues utilizing least-square misfortune work frequently have a precise insightful arrangement, however strategic relapse issues don’t. To comprehend the explanation, you should be comfortable with the idea of “convexity” in improvement. This line of examination will likewise enlighten why we should stay happy with “rough” arrangements in most AI issues.
Conclusion
Kindly don’t feel overpowered. There is a great deal of things to realize, there are amazing assets on the web. After an update on these points (which you likely concentrated on as an undergrad) and learning new ideas, i believe that you will be engaged to hear the secret music in your everyday data examination and AI projects. Also, that is a major jump toward turning into an astonishing data researcher.
You may even like: data science career in 2022