The Data Engineering Industry’s Future
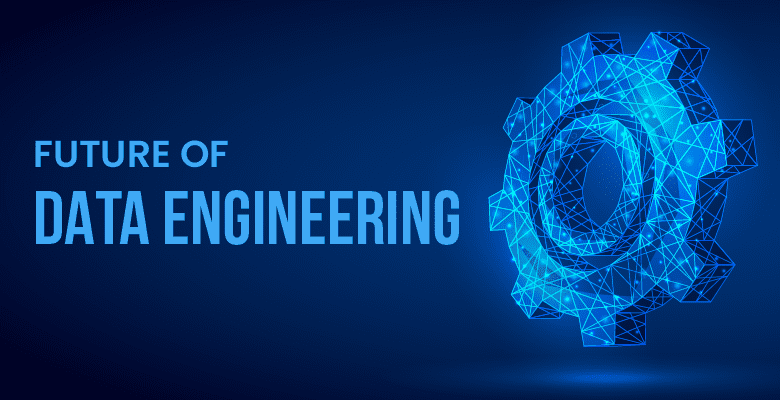
A Data Engineering Guide or data science in Malaysia reveals that while people frequently rely on data engineers‘ work — relying on Siri for quick solutions or being enchant by personalised recommendations or promotions — they frequently do not realise that these advanced tools are only accurate as a result of data engineers’ hard work. The examination of big data revealed that it is only as good as the Data Quality supplied by data engineers. “Data Engineering” is define in this handbook as “the process of collecting, translating, and validating data for analysis.”
Thus, in an ideal scenario, the data engineer establishes the data warehouse, data architecture, and data pipelines upon which the data scientist can perform complex analysis. They work in unison but never take on the function of the other.
The Changing Data Science And Data Engineering
The article The Changing Data Science And Data Engineering Tooling Environment cautions readers against the peril of combining data science and data engineering jobs inside a company. As with other technological disciplines, data technology fields should retain a clear boundary between “specialisations,” which is why individuals with pure Data Science skills or expertise should never be expect to fill the role of a data engineer and vice versa. The article’s message is clear: data science and data engineering are diametrically opposed activities that demand skill sets that are best suit for one or the other, but not for both.
So, You’re Interested in Becoming a Data Engineer? explains that whereas data scientists focus on large data analysis, data engineers establish the data architectures and data pipelines necessary for data analysis to occur.
Particularly now, with the integration of AI and machine learning with data technologies, data engineers are task with preparing data pipelines, whilst data scientists are task with performing data analysis purely for the purpose of extracting insights. As a result, the data engineer’s position has become as critical, if not more so, than the data scientist’s.
While future automation techniques may significantly reduce the demand for data engineers, they will continue to play a critical role in enterprise analytics teams. The issue with modern tool providers is that they frequently offer data scientists technological environments that actually require data engineers to be there and undertake initial data setup, cleaning, and preparation duties. We can only hope that this division of tasks becomes more apparent to company leaders and operators over time.
The Data Engineer’s Changing Role, data science in malaysia
With significant technological improvements, data engineering as a practise area is undergoing a sea change. The Internet of Things (IoT), serverless computing, hybrid cloud, artificial intelligence (AI), and machine learning have all had an impact on contemporary breakthroughs in data engineering (ML).
The Emergence and Future of the Data Engineer demonstrates how widespread usage of big data spawned the data engineer. However, the most significant advancement in data engineering occurred over the last eight years, owing to the growing automation of Data Science tools.
Modern corporate analytics platforms include fully or partially automated technologies for data collection, preparation, and cleansing in preparation for data scientists to examine. Nowadays, data scientists are no longer reliant on data engineers to furnish the data pipeline, as they were many years ago.
A single data engineer is adequate to assist a complete team of five or six data scientists/analysts in this case. While the data engineer is still necessary to optimise the data architecture and enable team members to work more efficiently, improved automation technologies are lowering the necessity for data engineers. Alternatively, are they? The Changing Role of the Data Engineer provides additional insight.
The importance of data engineer
According to a feature storey, global firms frequently “battle” to migrate from historical data to a “more flexible architecture.” This is where the data engineer’s work becomes important for a business’s digital readiness. 85% of respondents to a recent McKinsey poll indicated that they were “fairly effective” at meeting their enterprise data and analytics programmes’ goals.
In the blog post The Future of Data Engineering is the Convergence of Disciplines, Jasmine Tsai, Director of Engineering for Clover Health’s Data Platform, discusses her experience as a data engineer. She observes that “data engineering… in the not-too-distant future… (will) converge with other fields, particularly software engineering.” Tsai speculates that data engineering may reappear as a hybrid position very soon.
According to Data Engineering Advancements for 2019, “real-time analytics, streaming analytics, near real-time analytics, and complicated event processing” are all technical trends that apply to both data and software engineering. Thus, there are overlapping layers between software engineering and data engineering.
Not only are today’s data engineers proficient in a variety of cloud environments, but also in a variety of technologies “ranging from the Internet of Things (IoT) to Logical Data Warehouses (LDW).”
The Data Engineering Industry’s Future
With the transition from batch-based data movement and processing to real-time data movement and processing, a considerable shift toward “real-time data pipelines and real-time data processing systems” has occurred.
The data warehouse, with its incredible versatility to contain data marts, data lakes, or basic data sets as required, has risen in popularity in recent years. The book Emerging Trends in Data Engineering discusses how database streaming technology is laying the groundwork for the future of highly scalable, real-time corporate analytics.
The following four areas have been identify as future technological developments in data engineering:
Batch to Real Time: Systems for change data capture are increasingly displacing batch ETL, enabling database streaming. Traditional ETL functions are now performed in real time.
Connectivity between data sources and the data warehouse increased
Self-service analytics enabled by data engineering
Data Science functions can be automated
Hybrid data architectures that combine on-premises and cloud computing
Another significant development in recent data engineering technologies has been a focus on “data as it is” rather than on how and where it is kept. The move to real-time data processing has simplified data availability while increasing the difficulty of data processing. Data Management Trends in 2020 focuses on the Enterprise Data World Conference, which will have numerous notable speakers sharing their personal experiences with data engineering.
Source: data science course malaysia , data science in malaysia